概述
RNN (Recurrent Netural Network) 是用于处理序列数据的神经网络. 所谓序列数据, 即前面的输入和后面的输入有一定的联系.
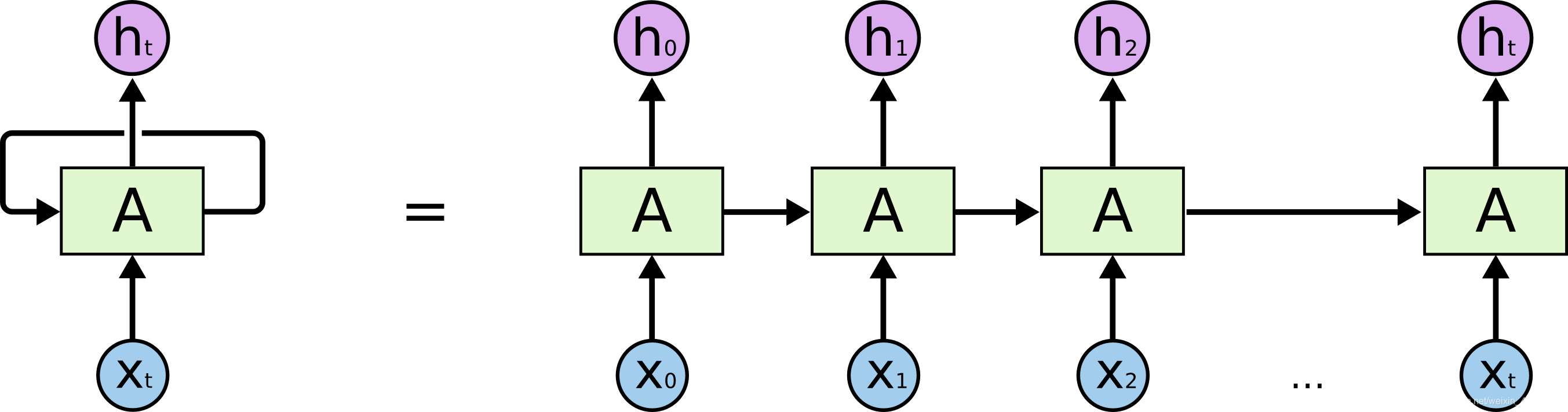
权重共享
传统神经网络:

RNN:

RNN 的权重共享和 CNN 的权重共享类似, 不同时刻共享一个权重, 大大减少了参数数量.
计算过程:
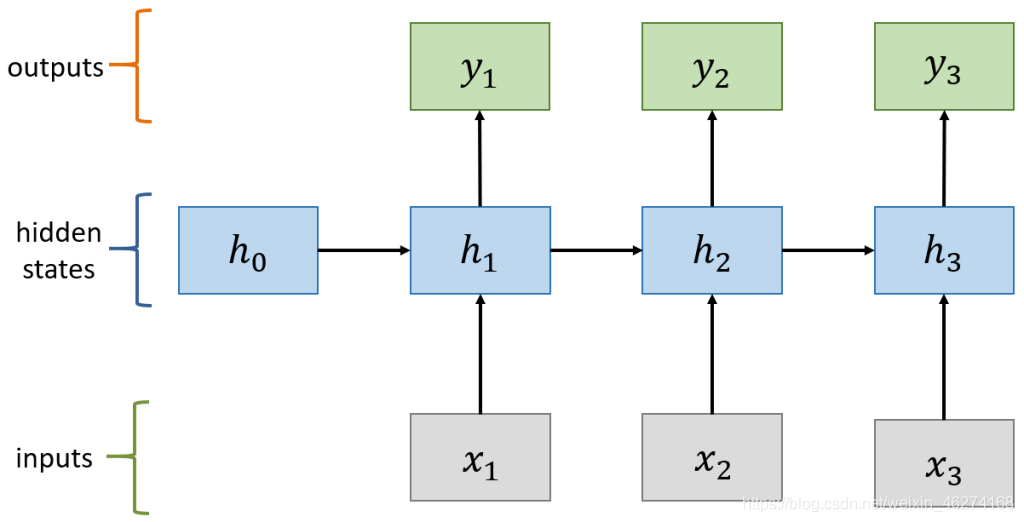
计算状态 (State)
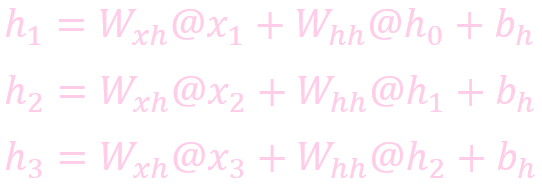
计算输出:

案例
数据集
IBIM 数据集包含了来自互联网的 50000 条关于电影的评论, 分为正面评价和负面评价.
RNN 层
class RNN(tf.keras.Model):
def __init__(self, units):
super(RNN, self).__init__()
# 初始化 [b, 64] (b 表示 batch_size)
self.state0 = [tf.zeros([batch_size, units])]
self.state1 = [tf.zeros([batch_size, units])]
# [b, 80] => [b, 80, 100]
self.embedding = tf.keras.layers.Embedding(total_words, embedding_len, input_length=max_review_len)
self.rnn_cell0 = tf.keras.layers.SimpleRNNCell(units=units, dropout=0.2)
self.rnn_cell1 = tf.keras.layers.SimpleRNNCell(units=units, dropout=0.2)
# [b, 80, 100] => [b, 64] => [b, 1]
self.out_layer = tf.keras.layers.Dense(1)
def call(self, inputs, training=None):
"""
:param inputs: [b, 80]
:param training:
:return:
"""
state0 = self.state0
state1 = self.state1
x = self.embedding(inputs)
for word in tf.unstack(x, axis=1):
out0, state0 = self.rnn_cell0(word, state0, training=training)
out1, state1 = self.rnn_cell1(out0, state1, training=training)
# [b, 64] -> [b, 1]
x = self.out_layer(out1)
prob = tf.sigmoid(x)
return prob
获取数据
def get_data():
# 获取数据
(X_train, y_train), (X_test, y_test) = tf.keras.datasets.imdb.load_data(num_words=total_words)
# 更改句子长度
X_train = tf.keras.preprocessing.sequence.pad_sequences(X_train, maxlen=max_review_len)
X_test = tf.keras.preprocessing.sequence.pad_sequences(X_test, maxlen=max_review_len)
# 调试输出
print(X_train.shape, y_train.shape) # (25000, 80) (25000,)
print(X_test.shape, y_test.shape) # (25000, 80) (25000,)
# 分割训练集
train_db = tf.data.Dataset.from_tensor_slices((X_train, y_train))
train_db = train_db.shuffle(10000).batch(batch_size, drop_remainder=True)
# 分割测试集
test_db = tf.data.Dataset.from_tensor_slices((X_test, y_test))
test_db = test_db.batch(batch_size, drop_remainder=True)
return train_db, test_db
完整代码
import tensorflow as tf
class RNN(tf.keras.Model):
def __init__(self, units):
super(RNN, self).__init__()
# 初始化 [b, 64]
self.state0 = [tf.zeros([batch_size, units])]
self.state1 = [tf.zeros([batch_size, units])]
# [b, 80] => [b, 80, 100]
self.embedding = tf.keras.layers.Embedding(total_words, embedding_len, input_length=max_review_len)
self.rnn_cell0 = tf.keras.layers.SimpleRNNCell(units=units, dropout=0.2)
self.rnn_cell1 = tf.keras.layers.SimpleRNNCell(units=units, dropout=0.2)
# [b, 80, 100] => [b, 64] => [b, 1]
self.out_layer = tf.keras.layers.Dense(1)
def call(self, inputs, training=None):
"""
:param inputs: [b, 80]
:param training:
:return:
"""
state0 = self.state0
state1 = self.state1
x = self.embedding(inputs)
for word in tf.unstack(x, axis=1):
out0, state0 = self.rnn_cell0(word, state0, training=training)
out1, state1 = self.rnn_cell1(out0, state1, training=training)
# [b, 64] -> [b, 1]
x = self.out_layer(out1)
prob = tf.sigmoid(x)
return prob
# 超参数
total_words = 10000 # 文字数量
max_review_len = 80 # 句子长度
embedding_len = 100 # 词维度
batch_size = 1024 # 一次训练的样本数目
learning_rate = 0.0001 # 学习率
iteration_num = 20 # 迭代次数
optimizer = tf.keras.optimizers.Adam(learning_rate=learning_rate) # 优化器
loss = tf.losses.BinaryCrossentropy(from_logits=True) # 损失
model = RNN(64)
# 调试输出summary
model.build(input_shape=[None, 64])
print(model.summary())
# 组合
model.compile(optimizer=optimizer, loss=loss, metrics=["accuracy"])
def get_data():
# 获取数据
(X_train, y_train), (X_test, y_test) = tf.keras.datasets.imdb.load_data(num_words=total_words)
# 更改句子长度
X_train = tf.keras.preprocessing.sequence.pad_sequences(X_train, maxlen=max_review_len)
X_test = tf.keras.preprocessing.sequence.pad_sequences(X_test, maxlen=max_review_len)
# 调试输出
print(X_train.shape, y_train.shape) # (25000, 80) (25000,)
print(X_test.shape, y_test.shape) # (25000, 80) (25000,)
# 分割训练集
train_db = tf.data.Dataset.from_tensor_slices((X_train, y_train))
train_db = train_db.shuffle(10000).batch(batch_size, drop_remainder=True)
# 分割测试集
test_db = tf.data.Dataset.from_tensor_slices((X_test, y_test))
test_db = test_db.batch(batch_size, drop_remainder=True)
return train_db, test_db
if __name__ == "__main__":
# 获取分割的数据集
train_db, test_db = get_data()
# 拟合
model.fit(train_db, epochs=iteration_num, validation_data=test_db, validation_freq=1)
输出结果:
Model: "rnn"
_________________________________________________________________
Layer (type) Output Shape Param #
=================================================================
embedding (Embedding) multiple 1000000
_________________________________________________________________
simple_rnn_cell (SimpleRNNCe multiple 10560
_________________________________________________________________
simple_rnn_cell_1 (SimpleRNN multiple 8256
_________________________________________________________________
dense (Dense) multiple 65
=================================================================
Total params: 1,018,881
Trainable params: 1,018,881
Non-trainable params: 0
_________________________________________________________________
None
(25000, 80) (25000,)
(25000, 80) (25000,)
Epoch 1/20
2021-07-10 17:59:45.150639: I tensorflow/compiler/mlir/mlir_graph_optimization_pass.cc:176] None of the MLIR Optimization Passes are enabled (registered 2)
24/24 [==============================] - 12s 294ms/step - loss: 0.7113 - accuracy: 0.5033 - val_loss: 0.6968 - val_accuracy: 0.4994
Epoch 2/20
24/24 [==============================] - 7s 292ms/step - loss: 0.6951 - accuracy: 0.5005 - val_loss: 0.6939 - val_accuracy: 0.4994
Epoch 3/20
24/24 [==============================] - 7s 297ms/step - loss: 0.6937 - accuracy: 0.5000 - val_loss: 0.6935 - val_accuracy: 0.4994
Epoch 4/20
24/24 [==============================] - 8s 316ms/step - loss: 0.6934 - accuracy: 0.5001 - val_loss: 0.6933 - val_accuracy: 0.4994
Epoch 5/20
24/24 [==============================] - 7s 301ms/step - loss: 0.6934 - accuracy: 0.4996 - val_loss: 0.6933 - val_accuracy: 0.4994
Epoch 6/20
24/24 [==============================] - 8s 334ms/step - loss: 0.6932 - accuracy: 0.5000 - val_loss: 0.6932 - val_accuracy: 0.4994
Epoch 7/20
24/24 [==============================] - 10s 398ms/step - loss: 0.6931 - accuracy: 0.5006 - val_loss: 0.6932 - val_accuracy: 0.4994
Epoch 8/20
24/24 [==============================] - 9s 382ms/step - loss: 0.6930 - accuracy: 0.5006 - val_loss: 0.6931 - val_accuracy: 0.4994
Epoch 9/20
24/24 [==============================] - 8s 322ms/step - loss: 0.6924 - accuracy: 0.4995 - val_loss: 0.6913 - val_accuracy: 0.5240
Epoch 10/20
24/24 [==============================] - 8s 321ms/step - loss: 0.6812 - accuracy: 0.5501 - val_loss: 0.6655 - val_accuracy: 0.5767
Epoch 11/20
24/24 [==============================] - 8s 318ms/step - loss: 0.6381 - accuracy: 0.6896 - val_loss: 0.6235 - val_accuracy: 0.7399
Epoch 12/20
24/24 [==============================] - 8s 323ms/step - loss: 0.6088 - accuracy: 0.7655 - val_loss: 0.6110 - val_accuracy: 0.7533
Epoch 13/20
24/24 [==============================] - 8s 321ms/step - loss: 0.5949 - accuracy: 0.7956 - val_loss: 0.6111 - val_accuracy: 0.7878
Epoch 14/20
24/24 [==============================] - 8s 324ms/step - loss: 0.5859 - accuracy: 0.8142 - val_loss: 0.5993 - val_accuracy: 0.7904
Epoch 15/20
24/24 [==============================] - 8s 330ms/step - loss: 0.5791 - accuracy: 0.8318 - val_loss: 0.5961 - val_accuracy: 0.7907
Epoch 16/20
24/24 [==============================] - 8s 340ms/step - loss: 0.5739 - accuracy: 0.8421 - val_loss: 0.5942 - val_accuracy: 0.7961
Epoch 17/20
24/24 [==============================] - 9s 378ms/step - loss: 0.5701 - accuracy: 0.8497 - val_loss: 0.5933 - val_accuracy: 0.8014
Epoch 18/20
24/24 [==============================] - 9s 361ms/step - loss: 0.5665 - accuracy: 0.8589 - val_loss: 0.5958 - val_accuracy: 0.8082
Epoch 19/20
24/24 [==============================] - 8s 353ms/step - loss: 0.5630 - accuracy: 0.8681 - val_loss: 0.5931 - val_accuracy: 0.7966
Epoch 20/20
24/24 [==============================] - 8s 314ms/step - loss: 0.5614 - accuracy: 0.8702 - val_loss: 0.5925 - val_accuracy: 0.7959
Process finished with exit code 0
到此这篇关于手把手教你使用TensorFlow2实现RNN的文章就介绍到这了,更多相关TensorFlow2实现RNN内容请搜索脚本之家以前的文章或继续浏览下面的相关文章希望大家以后多多支持脚本之家!
您可能感兴趣的文章:- tensorflow2.0实现复杂神经网络(多输入多输出nn,Resnet)
- windows系统Tensorflow2.x简单安装记录(图文)
- TensorFlow2基本操作之合并分割与统计
- 详解TensorFlow2实现前向传播
- Python强化练习之Tensorflow2 opp算法实现月球登陆器